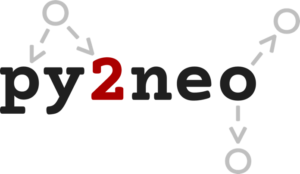
An OGM (Object-Graph Mapper) is to a graph database what an Object-Relational Mapper (ORM) is to a traditional RDBMS: a framework on which database-aware domain objects can be built.
The py2neo OGM centres its operation around the new
GraphObject
class. This acts as both a base class upon which domain objects can be defined and a manager for the underlying node and relationships that persist it.Take for example the Movie Graph that comes pre-packaged with Neo4j. We could model a
Person
from this dataset as below:class Person(GraphObject): __primarykey__ = "name" name = Property() born = Property()
Here, we define a
Person
class with two properties. Properties in Neo4j have no fixed type so there’s less to define than there would be for a SQL field in a typical ORM. We’re also using the same names for the class attributes as we do for the underlying properties:
name
and born
. If necessary, these could be redirected to a differently-named property with an expression such as Property(name="actual_name")
.Lastly, we define a
__primarykey__
. This tells py2neo which property should be treated as a unique identifier for push and pull operations. We could also define a __primarylabel__
although by default, the class name Person
will be used instead.All of this means that behind the scenes, the node for a specific
Person
object could be selected using a Cypher statement such as:MATCH (a:Person) WHERE a.name = {n} RETURN a
Broadening out a little, if we wanted to model both
Person
and Movie
from that same dataset, as well as the relationships that connect them, we could use the following:class Movie(GraphObject): __primarykey__ = "title" title = Property() tagline = Property() released = Property() actors = RelatedFrom("Person", "ACTED_IN") directors = RelatedFrom("Person", "DIRECTED") producers = RelatedFrom("Person", "PRODUCED") class Person(GraphObject): __primarykey__ = "name" name = Property() born = Property() acted_in = RelatedTo(Movie) directed = RelatedTo(Movie) produced = RelatedTo(Movie)
This introduces two new attribute types:
RelatedTo
and RelatedFrom
. These define sets of related objects that are all connected in a similar way. That is, they share a common start or end node plus a common relationship type.Take for example
acted_in = RelatedTo(Movie)
. This describes a set of related Movie
nodes that are all connected by an outgoing ACTED_IN
relationship. Note that like the property name above, the relationship type defaults to match the attribute name itself, albeit upper-cased. Conversely, the corresponding reverse definition, actors = RelatedFrom("Person", "ACTED_IN")
, specifies the relationship name explicitly as this differs from the attribute name.So how do we work with these objects? Let’s say that we want to pluck Keanu Reeves from the database and link him to the timeless epic Bill & Ted’s Excellent Adventure (sadly omitted from the original graph). First we need to select the actor using the
GraphObject
class method select
via the Person
subclass. Then, we can build a new Movie
object, add this to the set of movies acted_in
by the talented Mr Reeves and finally push everything back into the graph. The code looks something like this:keanu = Person.select(graph, "Keanu Reeves").first() bill_and_ted = Movie() bill_and_ted.title = "Bill & Ted's Excellent Adventure" keanu.acted_in.add(bill_and_ted) graph.push(keanu)
All related objects become available to instances of their parent class through a set-like interface, which offers methods such as add and
remove
. When these details are pushed back into the graph, the OGM framework automatically builds and runs all the necessary Cypher to make this happen.More complex selections are possible through the
select
method as well. The where
method can make use of any expression that can be used in a Cypher WHERE
clause. For example, to output the names of every actor whose name starts with ‘K’, you could use:for person in Person.select(graph).where("_.name =~ 'K.*'"): print(person.name)
Note that the underscore character is used here to refer to the node or nodes being matched.
There’s a lot more information available in the py2neo documentation and there’s also a demo application in the GitHub repository that shows how this all comes together in a mini movie browser (screenshot below).
As always, if you have any questions about py2neo or the official drivers, I’ll try my best to help. My contact details can be found somewhere on this page probably.
Want to learn more about graph databases and Neo4j? Click below to register for one of our online training classes, Introduction to Graph Databases or Neo4j in Production and catch up to speed with graph database technology.